Artificial intelligence is becoming an increasingly important part of our lives. However, when it comes to understanding human language, technology still isn't at the point where it can give us all the answers.
Since the 1950s, the computer and language have been working together from obtaining simple input to complex texts. It was Alan Turing who performed the Turing test to know if machines are intelligent enough or not.
That's why companies are using natural language processing to extract information from text.
With AI and machine learning (ML), NLU(natural language understanding), NLP ((natural language processing), and NLG (natural language generation) have played an essential role in understanding what user wants.
Furthermore, NLU and NLG are parts of NLP that are becoming increasingly important. These technologies use machine learning to determine the meaning of the text, which can be used in many ways.
So, What is the difference between NLU and NLP? To understand this, we first need to know what each term stands for and clarify any ambiguities.
In this blog article, we have highlighted the difference between NLU and NLP and understand the nuances.
What is Natural Language Processing?
NLP or natural language processing is evolved from computational linguistics, which aims to model natural human language data.
Also, NLP processes a large amount of human data and focus on use of machine learning and deep learning techniques.
It is commonly used in computer science, information systems, linguistics, communications, and philosophy.
NLP has many subfields, including computational linguistics, syntax analysis, speech recognition, machine translation, and more.

Natural language processing works by taking unstructured text and converting it into a correct format or a structured text. It works by building the algorithm and training the model on large amounts of data analyzed to understand what the user means when they say something.
It works by taking and identifying various entities together (named entity recognition) and identification of word patterns. The word patterns are identified using methods such as tokenization, stemming, and lemmatization.
NLP undertakes various tasks such as parsing, speech recognition, part-of-speech tagging, and information extraction.
In the real world, NLP is used for text summarization, sentiment analysis, topic extraction, named entity recognition, parts-of-speech tagging, relationship extraction, stemming, text mining, machine translation, and automated question answering, as well as ontology population, language modelling, and any other language-related task.
What is natural language understanding?
NLU is a subset of natural language processing that uses the semantic analysis of text to understand the meaning of sentences.
It's possible that the same text can have many meanings, that different words can have the same meaning, or that the meaning can change depending on the situation.
NLU algorithms process text from different sources using computational methods to reach some understanding of an input text, which is as simple as understanding what a sentence says or as complex as understanding the dialogue between two people.

It converts your text into a machine-readable format.
For example, it is the process of recognizing and understanding what people say in social media posts.
So, NLU uses computational methods to understand the text and produce a result.
NLU can be used in many different ways, including understanding dialogue between two people, understanding how someone feels about a particular situation, and other similar scenarios.
There are namely three linguistic levels to understand NLU:
- Syntax: This is the process of understanding how sentences are constructed and if the grammar is used correctly. For example, to understand if a sentence makes sense, it must be considered in context and its syntax analyzed.
- Semantics: When we look at the text that contains contextual meaning details such as tone of voice or word choice between two people. These pieces of data can also be used for an NLU algorithm to produce results from all possible contexts in which the same piece of spoken
- Pragmatic analysis: It helps understand the context and what the text is trying to achieve.
- Word sense disambiguation is the process of determining the meaning of words in sentences. It gives a word meaning based on its context.
Do we need both NLP & NLU?
Yes, NLU and NLP work together. Further, the terms NLP and NLU are used interchangeably.
They work together to create more complex NLP models. A common example of this is sentiment analysis, which uses both NLP and NLU algorithms in order to determine the emotional meaning behind a text.
While both NLP and NLU are related, they are different in their aims. However, NLP & NLU are different data mining techniques.
Natural language understanding is the process by which a machine interprets a user's query or request and uses sentiment analysis, part-of-speech tagging, subject classification, and other machine learning techniques to deduce the user's intent.
NLP models are designed to describe the meaning of sentences whereas NLU models are designed to describe the meaning of the text in terms of concepts, relations and attributes.
NLP vs NLU: What’s the difference?
The major difference between the NLU and NLP is that NLP focuses on building algorithms to recognize and understand natural language, while NLU focuses on the meaning of a sentence.
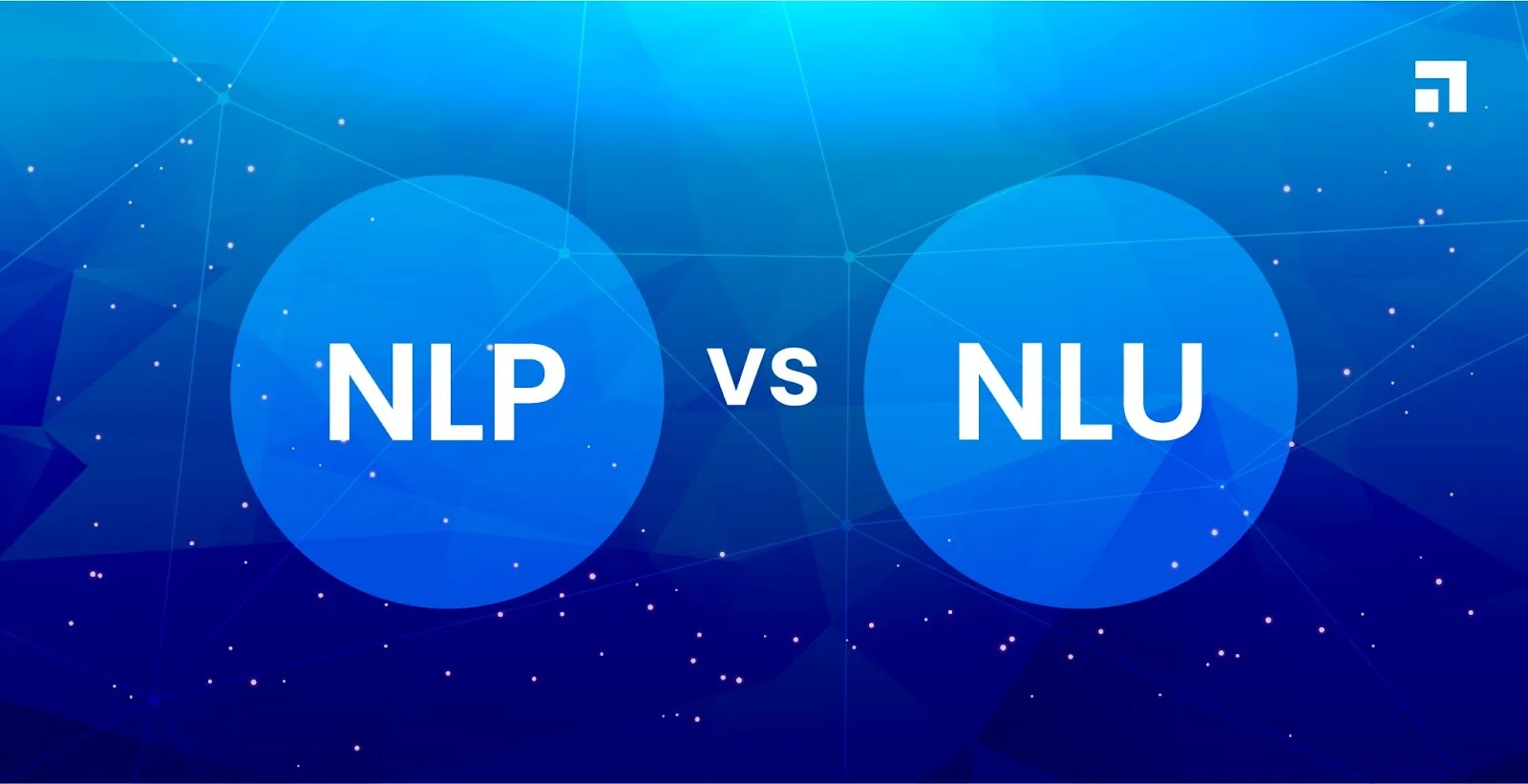
Another difference is that NLP breaks and processes language, while NLU provides language comprehension.
Both NLU and NLP use supervised learning, which means that they train their models using labelled data. However, the difference between them is in how it's done.
Another difference between NLU and NLP is that NLU is focused more on sentiment analysis. Sentiment analysis involves extracting information from the text in order to determine the emotional tone of a text.
Natural language processing and natural language understanding language are not just about training a dataset. The computer uses NLP algorithms to detect patterns in a large amount of unstructured data.
NLU recognizes that language is a complex task made up of many components such as motions, facial expression recognition etc. Furthermore, NLU enables computer programmes to deduce purpose from language, even if the written or spoken language is flawed.
NLP & NLU use cases
Only 20% of data on the internet is structured data and usable for analysis. The rest 80% is unstructured data, which can't be used to make predictions or develop algorithms.
That's where NLP & NLU techniques work together to ensure that the huge pile of unstructured data is made accessible to AI. Both NLP& NLU have evolved from various disciplines like artificial intelligence, linguistics, and data science for easy understanding of the text.

Machines help find patterns in unstructured data, which then help people in understanding the meaning of that data. This is how NLP & NLU use cases are being implemented today.
Some common use cases of NLP & NLU are:
1. Personal assistants (Siri, Alexa and Google Home)
2. Image and video recognition
3. Content moderation
4. Sentiment Analysis
NLP and NLU together
NLP and NLU work together to help solve a number of problems. They both offer similar benefits, which makes them an excellent choice for any company looking to leverage AI.
The two pillars of NLP are syntactic analysis and semantic analysis.
To help you understand the advantages of NLP and NLU together, let's take a look at some use cases:
In 2017, LinkedIn expanded its AI capabilities by integrating NLP & NLU into their platform.
This allowed it to provide relevant content for people who were interested in specific topics. This allowed LinkedIn to improve its users' experience and enable them to get more out of their platform.
NLP & NLU works in various industries together:
1. Finance & Banking
2. Media & Entertainment
3. Government & Healthcare
4. Retail & Consumer Goods
5. Automotive & Transportation
What is the future of natural language?
Pursuing the goal to create a chatbot that can hold a conversation with humans, researchers are developing chatbots that will be able to process natural language.
The ultimate goal is to create an intelligent agent that will be able to understand human speech and respond accordingly.
That's why NLU, NLP and NLG work together. In fact, A combination of NLU and NLG gives an NLP system.
However, there are still many challenges ahead for NLP & NLU in the future. One of the main challenges is to teach AI systems how to interact with humans.
Machine Learning (ML) AI: Data Training
In order to make the most of your machine learning systems, it’s important to understand how ML works. There are a few different stages for training an ML system: data acquisition, feature engineering and model building.
However, Computers use much more data than humans do to solve problems, so computers are not as easy for people to understand as humans are. Even with all the data that humans have, we are still missing a lot of information about what is happening in our world.
Thus, we need AI embedded rules in NLP to process with machine learning and data science.
NLP vs NLU Summary
With technological advancements, NLP and NLU are used in a lot of different ways, but they're usually interlinked. If you're building an app that will have a chat function, then the two terms should be familiar to you.
Both NLU & NLP play a vital role in understanding the human language.
The difference between them is that NLP can work with just about any type of data, whereas NLU is a subset of NLP and is just limited to structured data. In other words, NLU can use dates and times as part of its conversations, whereas NLP can't.
It's also important to remember that although both NLP and NLU are used for conversational apps, they have their own uses as well.
FAQs
Q. Which one is better: NLU or NLP?
There is no definitive answer as to which one is better: NLU or NLP. However, both of these types of training offer great benefits for individuals looking to improve their communication skills.
NLP stands for neuro-linguistic programming, and it is a type of training that helps people learn how to change the way they think and communicate in order to achieve their goals.
NLU stands for natural language understanding, and it is a type of training that helps people learn how to understand and interpret the language being used around them.
Both types of training are highly effective in helping individuals improve their communication skills, but there are some key differences between them. NLP offers more in-depth training than NLU does, and it also focuses on teaching people how to use neuro-linguistic programming techniques in their everyday lives.
Q. What is NLU in Machine Learning?
Natural language understanding is a subset of machine learning that helps machines learn how to understand and interpret the language being used around them. This type of training can be extremely beneficial for individuals looking to improve their communication skills, as it allows machines to process and comprehend human speech in ways that humans can.
Q. Is NLG a subset of NLP?
Natural language processing (NLP), natural language understanding (NLU), and natural language generation (NLG) are all related but different issues. NLU and NLG are components of NLP at a high level.
Q. Why is NLU harder than NLG?
NLU deciphers the data based on grammatical rules, the context in which it was said, and determines intent and entities. Text is generated by NLG based on structured data.